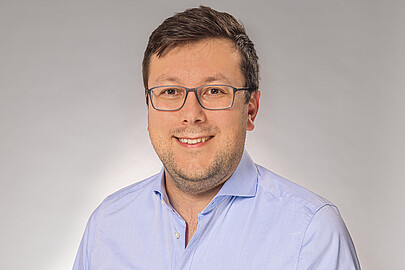
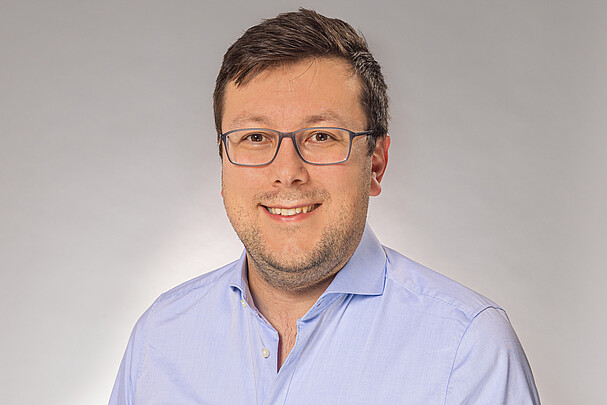
30167 Hannover
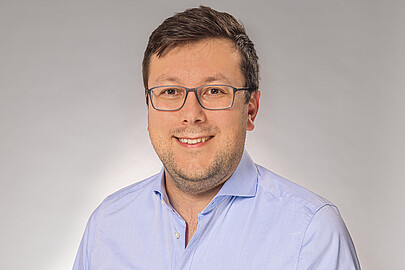
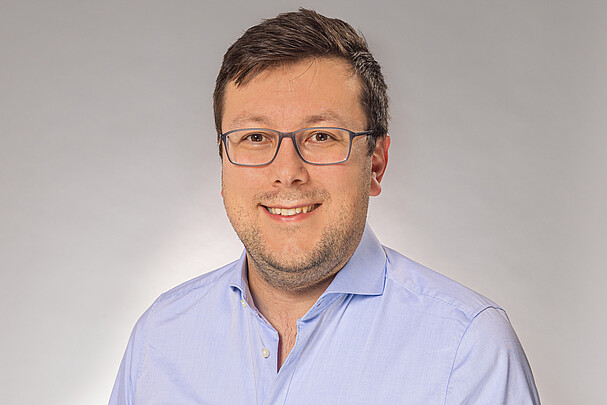
My research is centered around automated machine learning (AutoML) and related topics such as meta-learning, hyperparameter optimization, algorithm configuration and algorithm selection, as well as supervised learning methods. Specifically, I am interested in methods for multi-label classification. Beyond that, my research interests are widespread and include uncertainty quantification, evolutionary machine learning, machine learning in IT security, part of speech tagging, service-oriented software architectures, and (co-)active learning.
Research Interests
- Interactive and Explainable AutoML
- Green AutoML
- Meta-Learning
- Hyperparameter Optimization
- Algorithm Configuration and Algorithm Selection
- Multi-Label Classification
- (Co-)Active learning
Further research interests:
- Uncertainty quantification
- Evolutionary machine learning
- Machine learning in IT security
- Part-of-speech tagging
- Service-oriented software architectures
Curriculum Vitae
-
Working Experience
2024 - Present
Postdoctoral Researcher, Leibniz University Hannover2023 - 2024
Expert Consultant for Machine Learning, Fraunhofer IEM2022 - 2024
Transfer Coordinator for Education, Munich Center for Machine Learning2021 - 2024
Postdoctoral Researcher, LMU Munich2017 - 2021
Doctoral Researcher, Paderborn University -
Education
2017 - 2021
Ph.D. Student (Dr. rer. nat) supervised by Prof. Dr. Eyke Hüllermeier, Paderborn University2015 - 2017
Master of Science, Computer Science, Paderborn University2011 - 2015
Bachelor of Science, Computer Science, Paderborn University -
Selected Awards
2022
Outstanding reviewer at NeurIPS 2022.2021
Outstanding reviewer at ICML 2021.2020
Outstanding reviewer at ICML 2020.2020
Frontier Prize for the most visionary contribution at the International Symposium on Intelligent Data Analysis, 2020.2019
Young author award at the Computational Intelligence Workshop, Dortmund, 2019.2017
Young author award at the Computational Intelligence Workshop, Dortmund, 2017.Best paper award for the SBSE/ACO-SI track at the Genetic and Evolutionary Computation Conference (GECCO), 2017.
-
Memberships
2020 - Present
Member of the Benchmarking network2019 - Present
Core developer of OpenML2019 - Present
Member of the COSEAL network - Social Media
Publications
Zeige Ergebnisse 1 - 30 von 49