Overview
Semester | Summer |
ECTS | 5 |
Level | Bachelor |
Description
The course will be held in English.
Enlisting is done exclusively via studip on a first-come-first-serve basis. There is a limit of 100 Students.
In the Era of Big Data, one of the emerging requirements for any scientist is the ability to effectively and critically work with data, i.e., collect and extract data, create surveys, transform the data, apply mathematical models on the data, and visualize the important aspects. In fact, the Society of Computer Science (Gesellschaft der Informatik) has coined the term data literacy to describe various competencies in this regard. In the same spirit, the goal of this course is to teach non-computer scientists the foundational concepts of data science. Students will learn to analyze data for the purpose of understanding and describing real-world phenomena. The students will obtain skills in data-centric programming and statistical inference. Furthermore, the students will gain hands-on experience on daily challenges of a data scientist with best practice approaches for data collection and preparation. Finally, we will discuss ethical and social aspects of data science. The course consists of a standard lecture and lab work. During the lecture the important concepts are introduced. In the lab sessions, students will be guided in practical programming exercises. In addition, the students receive bi-weekly assignments that follow-up on the lab exercises. The successful participation in the assignments is a pre-requisite to take part in the final written exam.
Recommended pre-requisites
- Probability theory
- Data structures and algorithms
- Foundations of data bank systems
Lecturer
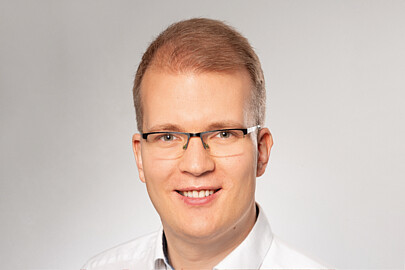
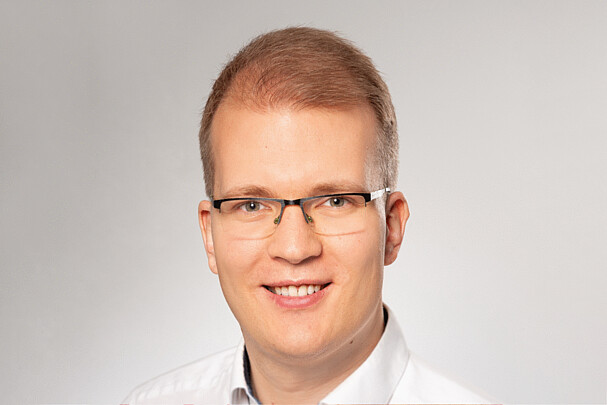
30167 Hannover
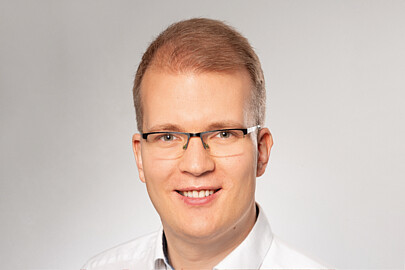
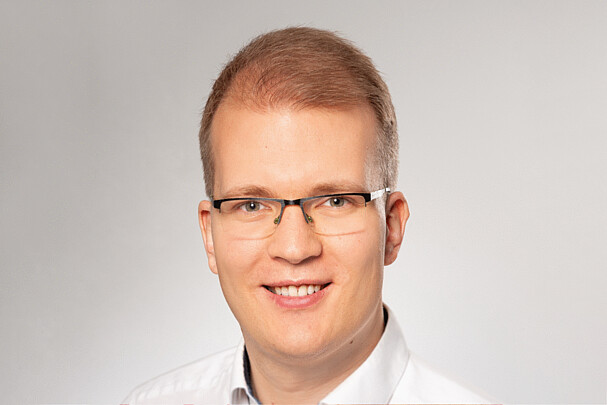
Topics
- Data Sampling and Probability
- data preparation
- visualizations
- Intro to Modeling
- Simple Linear Regression + OLS
- feature engineering
- Bias and Variance
- Cross-Validation, Regularization and AutoML
- classification
- Inference for Modeling
- Conclusion and Ethics
Literature
The full list of literature can be found on the StudentIP course page. Recommended books to accompany the lecture are:
Computational and Inferential Thinking: The Foundations of Data Science 2nd Edition by Ani Adhikari, John DeNero, David Wagner.
- Principles and Techniques of Data Science by Sam Lau, Joey Gonzalez, and Deb Nolan.